No Traffic Tech
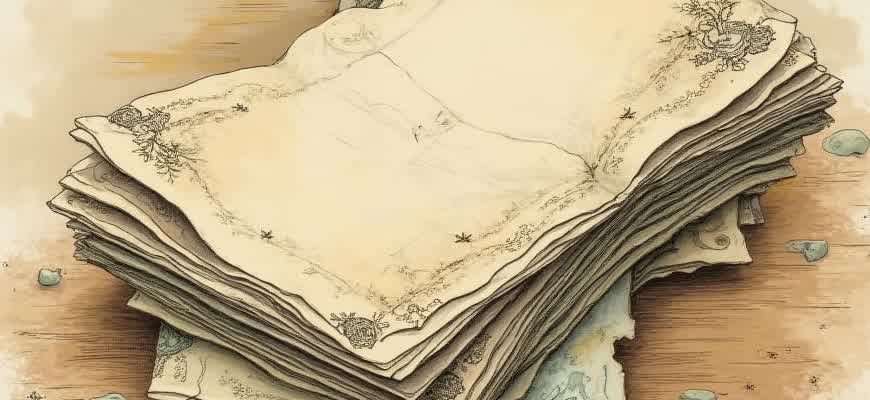
Urban congestion has become a major bottleneck for modern cities, leading to lost productivity, increased emissions, and declining quality of life. As traditional infrastructure upgrades struggle to keep pace, a new wave of smart mobility systems is emerging, targeting the root causes of traffic buildup with precision technology.
- AI-driven traffic signal optimization
- Autonomous shuttle networks on fixed urban loops
- Integrated micro-mobility hubs with real-time availability tracking
Key Insight: Replacing reactive traffic systems with predictive, sensor-integrated networks can reduce urban congestion by up to 40%.
Decentralized commuting strategies are also reshaping how people move through cities. These approaches redistribute transportation load and eliminate pressure from central roads and peak-time corridors.
- Staggered work schedules enforced by enterprise mobility apps
- Community-based carpooling with AI-matched routes
- Dedicated lanes for delivery drones and autonomous cargo bots
Technology | Impact | Implementation |
---|---|---|
Real-time congestion mapping | Prevents route overload | Mobile apps, traffic cameras |
Smart curb management | Streamlines last-mile logistics | IoT-enabled parking zones |
Automated traffic enforcement | Reduces gridlock from violations | AI-powered roadside units |
Reducing Idle Time for Delivery Vehicles Through Real-Time Traffic Bypass
Minimizing stationary periods for logistics fleets requires bypassing high-congestion zones using adaptive routing. Delivery trucks often lose valuable operational time due to unanticipated traffic buildup, particularly in urban centers during peak hours. Incorporating live navigation adjustments enables smoother progress and fewer delays at intersections and bottlenecks.
Intelligent rerouting systems analyze current traffic patterns and predict congestion points, allowing fleet managers to divert drivers before they encounter delays. This minimizes engine idle time and contributes to reduced fuel consumption and emissions. Real-time updates also improve estimated time of arrival (ETA) accuracy, enhancing service reliability.
Key Components of Dynamic Routing Implementation
- Sensor Integration: Use of GPS and IoT sensors to detect slowdowns and queue formation.
- Data Aggregation: Continuous data intake from city infrastructure, traffic cameras, and weather APIs.
- Route Optimization Algorithms: Automated decision-making tools that suggest alternate paths instantly.
Fleets equipped with dynamic rerouting systems report up to 30% reduction in idle time and a 15% drop in fuel use within metropolitan areas.
Factor | Without Bypass | With Real-Time Rerouting |
---|---|---|
Average Idle Time (per route) | 18 minutes | 11 minutes |
Fuel Wasted | 2.3 liters | 1.4 liters |
ETA Accuracy | 72% | 91% |
- Install connected vehicle telemetry hardware across all delivery units.
- Integrate city-wide traffic data into the fleet management platform.
- Activate automatic rerouting protocols based on real-time traffic input.
Analyzing Investment Efficiency Through Route Patterns and Commute Records
Modern transportation initiatives demand precise tools to measure effectiveness. By examining historical commute records and mapping vehicle movement across typical routes, organizations can assess where infrastructure or technology investments yield real improvements in journey consistency and speed.
Transport managers and urban planners increasingly turn to structured route logs and timestamped travel entries to quantify operational gains. These datasets help isolate the impact of interventions–such as signal optimization or adaptive routing–on actual commute durations and route reliability.
Key Evaluation Methods
- Baseline Travel Benchmarking: Analyze pre-intervention route durations to set reference points for ROI comparison.
- Time Variance Tracking: Use logs to identify fluctuations in travel time before and after applying changes.
- Route Deviation Metrics: Measure how often and why vehicles divert from optimized paths.
Consistent reduction in average route duration–even by 10%–translates directly into lower operational costs and higher throughput.
Metric | Before Optimization | After Optimization |
---|---|---|
Average Trip Duration | 36 minutes | 28 minutes |
Fuel Consumption per Trip | 4.2 liters | 3.5 liters |
Route Adherence Rate | 78% | 91% |
- Extract timestamped route data from GPS logs.
- Segment routes by time of day and vehicle type.
- Compare performance indicators across defined periods.
Focused analysis of travel logs enables not just cost savings but evidence-based planning for future infrastructure projects.
Common Implementation Pitfalls and How to Avoid Them
Deploying sensor-based traffic-free technologies in urban environments often encounters technical and organizational missteps. These issues can slow down project timelines, increase costs, or even render systems ineffective. Understanding and anticipating these challenges is critical for successful integration.
Below are specific mistakes frequently observed during rollout phases and clear steps to mitigate each. Decision-makers, engineers, and urban planners should evaluate these points before proceeding with deployment.
Key Failures and Practical Prevention Methods
- Overreliance on Static Data: Many systems operate on pre-programmed assumptions about vehicle behavior and population flow, making them ineffective during anomalies like events or roadworks.
- Poor Integration with Legacy Infrastructure: Attempting to overlay advanced automation on outdated systems without compatibility testing often leads to data mismatches and functionality gaps.
- Neglecting Real-Time Feedback Loops: Systems that don’t include real-time user input (drivers, cyclists, pedestrians) can’t adapt to on-the-ground reality.
Strong planning must include a dynamic layer of live data interpretation and response – this is the backbone of effective implementation.
- Audit Existing Infrastructure: Conduct technical assessments to identify bottlenecks in communication between new and existing systems.
- Simulate Edge Cases: Use digital twins or simulation environments to test failure points under high-stress or irregular conditions.
- Implement Modular Architecture: Design systems in swappable layers to allow for incremental upgrades and debugging.
Issue | Consequence | Recommended Solution |
---|---|---|
Insufficient interoperability | System silos and inconsistent responses | Use open APIs and cross-platform protocols |
Low public engagement | Noncompliance or misreporting | Incorporate user feedback channels via mobile apps |