Fundamentals of Traffic Flow
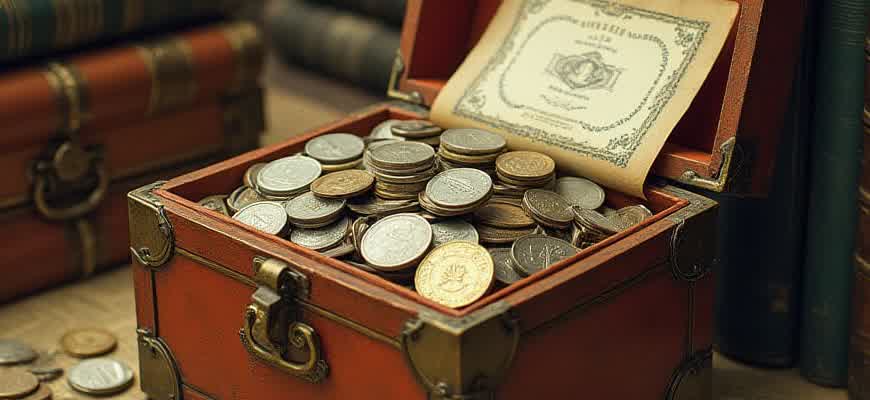
Understanding how vehicles interact on roadways involves analyzing specific parameters that govern flow conditions. These metrics are interdependent and provide a framework for predicting and managing traffic performance.
- Vehicle Density (k): Number of vehicles per unit length of roadway, usually vehicles per kilometer.
- Traffic Intensity (q): Rate at which vehicles pass a point on the road, measured in vehicles per hour.
- Mean Speed (v): Average speed of all vehicles, derived from spot or time-mean observations.
The fundamental relation is expressed as: q = k × v, illustrating how flow is a product of density and velocity.
Flow regimes can be categorized based on operational characteristics and vehicle interactions:
- Free-flow Conditions: Low density, minimal interaction, and speeds close to desired values.
- Unstable Flow: Moderate density, with growing influence of leading vehicles on speed.
- Congested Regime: High density, stop-and-go motion, and reduced throughput.
Flow State | Density (veh/km) | Average Speed (km/h) | Flow Rate (veh/h) |
---|---|---|---|
Free-flow | 0–20 | 80–120 | 1600–2000 |
Unstable | 20–50 | 40–80 | 2000–3000 |
Congested | 50–100+ | 0–40 | <2000 |
Influence of Vehicle Concentration on Urban Commute Duration
In metropolitan areas, the number of vehicles occupying a given stretch of road–known as vehicle concentration–directly affects how long it takes for a commuter to reach their destination. As more cars enter a road segment, the spacing between them shrinks, forcing drivers to reduce speed and react more frequently to surrounding movements.
This buildup leads to irregular flow patterns and extended delays, especially during peak periods. The progression from free-flow conditions to a state of saturation can be illustrated through empirical models that correlate average speed with vehicle presence per kilometer of lane.
Key Consequences of High Vehicle Presence
A road operating at or near its capacity loses predictability–travel times become volatile and prone to rapid escalation with small increases in vehicle volume.
- Reduced average velocity: Vehicles move slower as space gaps tighten.
- Increased stop-and-go behavior: Frequent braking and acceleration cycles occur.
- Elevated travel time variability: Commuters experience inconsistent durations.
- Light traffic: Smooth travel, minimal interference.
- Moderate traffic: Some delays, speed fluctuations.
- Heavy traffic: Long queues, frequent halts.
Vehicle Density (vehicles/km) | Average Speed (km/h) | Typical Delay (minutes/km) |
---|---|---|
10 | 55 | 0.5 |
30 | 35 | 2.0 |
60 | 15 | 5.5 |
Predicting Bottlenecks with Discontinuity Propagation
Traffic discontinuities, often referred to as kinematic waves, represent abrupt changes in vehicle density and speed. These waves can move either forward or backward relative to the flow of traffic. By analyzing their direction and speed, it becomes possible to identify areas where congestion will likely intensify or dissipate.
Backward-propagating waves typically signal the formation of traffic jams. When the inflow of vehicles exceeds the outflow at a specific point–such as an on-ramp or lane reduction–a compression wave forms and moves upstream. Engineers use this dynamic to determine the potential extent and severity of slowdowns before they manifest fully.
Wave Behavior Analysis
- Forward waves: Occur during acceleration or dispersal of vehicles.
- Backward waves: Indicate vehicle accumulation, often caused by reduced capacity ahead.
The velocity of a wave is calculated using the slope of the flow-density curve: v = (q2 - q1) / (k2 - k1), where q is traffic flow and k is density.
Scenario | Wave Type | Expected Effect |
---|---|---|
Sudden braking due to obstacle | Backward | Congestion buildup upstream |
End of bottleneck (e.g., added lane) | Forward | Flow normalization |
- Identify density changes using real-time sensor data.
- Determine wave speed and direction.
- Predict future congestion zones and implement control measures.
Calibrating Speed-Flow Models for Real-Time Traffic Simulation
Accurate tuning of velocity–density relationships is essential for reliable traffic flow forecasting systems. In real-time applications, models must respond dynamically to changing road conditions, which requires a precise match between measured and simulated parameters. This calibration process involves fitting mathematical relationships that reflect driver behavior under various congestion levels.
Key steps include collecting high-resolution detector data, identifying optimal model parameters through optimization algorithms, and validating performance using error metrics like RMSE or MAPE. These steps ensure that the model output aligns closely with observed traffic states, enhancing its predictive reliability for live monitoring systems.
Steps in Parameter Tuning Process
- Gather loop detector or GPS-based traffic data at short intervals (e.g., 5 seconds).
- Select an appropriate empirical model (e.g., Greenshields, Underwood, or Newell).
- Apply nonlinear regression or metaheuristic optimization (e.g., genetic algorithms).
- Compare simulated flow-speed outputs with field measurements.
- Iteratively refine the model to minimize prediction error.
Note: Poor calibration leads to significant deviations in congestion propagation and queue length estimations, which undermines control strategies like ramp metering or variable speed limits.
Model Type | Primary Parameter | Common Use Case |
---|---|---|
Greenshields | Free-flow speed | Urban arterials |
Underwood | Speed decay rate | Freeways |
Newell | Backward wave speed | Congestion modeling |
- Real-time integration demands recalibration at regular intervals.
- Sensor noise and latency must be filtered to prevent model drift.
- Multi-lane data fusion improves accuracy in complex road networks.
Choosing Between Microscopic and Macroscopic Traffic Models
When simulating traffic dynamics, analysts must select an appropriate modeling approach based on the required resolution and available data. High-resolution frameworks represent each vehicle and driver behavior individually, capturing phenomena such as lane-changing, car-following, and reaction delays. In contrast, lower-resolution models treat traffic as a continuous flow, similar to fluid dynamics, focusing on aggregate variables like density, flow rate, and average speed.
The decision between these frameworks depends on the specific use case, computational resources, and scale of the traffic system being analyzed. For local interventions, such as signal optimization at intersections, detailed models offer precise results. For large-scale planning, where the interest lies in general trends across a network, simplified models provide faster computation with acceptable accuracy.
Comparison of Modeling Approaches
Criteria | High-Resolution Models | Low-Resolution Models |
---|---|---|
Granularity | Individual vehicles | Traffic streams |
Computation Time | High | Low |
Data Requirement | Detailed trajectory data | Aggregate sensor data |
Application Scale | Urban corridors, intersections | City-wide, regional networks |
Note: High-resolution modeling is essential for studying driver interactions and autonomous vehicle behavior, while low-resolution models are ideal for strategic planning and congestion forecasting.
- Use detailed models when analyzing individual behaviors, such as stop-and-go waves or collision risks.
- Use simplified models when focusing on network-wide throughput or long-term policy evaluation.
- Identify the scale and scope of the traffic problem.
- Match the modeling resolution to the data availability and computational budget.
- Select the framework that aligns with the desired level of detail and performance metrics.
Utilizing Queue Models to Optimize Intersection Throughput
Understanding how vehicles accumulate and disperse at traffic signals allows for more precise management of urban traffic. By modeling intersections as queue systems, engineers can analyze arrival and departure rates, identifying when congestion is likely to occur and how long it persists. This analytical approach provides measurable insights into the efficiency of traffic signal timing and lane usage.
Queue models, especially those involving stochastic arrival patterns, help simulate real-world conditions where vehicle flow is inconsistent. Using probabilistic distributions for arrivals and deterministic or service-based departure times, these models guide decisions such as cycle length, phase splits, and turn lane allocation to alleviate vehicle stacking at peak periods.
Core Applications of Queueing Models at Signalized Crossings
- Evaluating average delay per vehicle using M/M/1 and M/D/1 models.
- Estimating queue length and clearance time during red phases.
- Prioritizing signal allocation based on queue spillback risks.
Note: When the vehicle arrival rate exceeds the departure rate for a prolonged time, the system reaches saturation, leading to exponential queue growth.
- Measure real-time vehicle arrival rate (λ) and service rate (μ).
- Apply Little’s Law: L = λW, where L is average queue length and W is average wait time.
- Adjust signal phase timing dynamically to balance demand and capacity.
Model | Assumptions | Use Case |
---|---|---|
M/M/1 | Poisson arrivals, exponential service | Random flows at minor intersections |
M/D/1 | Poisson arrivals, fixed service time | Signalized intersections with fixed green intervals |
D/D/1 | Deterministic arrivals and service | High-precision signal timing optimization |
Decoding the Core Diagram for Enhancing Traffic Efficiency
Understanding the relationship between vehicle density and movement rate is crucial for managing highway and urban congestion. The curve that plots this relationship provides actionable insights for adjusting traffic signals, lane allocation, and ramp metering in real time.
This curve illustrates how throughput varies with concentration levels on the road. Initially, increasing the number of vehicles boosts total flow, but beyond a certain threshold, added vehicles reduce average speed and disrupt equilibrium.
Key Concepts Illustrated in the Flow-Density Graph
- Free-flow zone: Low density and high velocity, indicating underused infrastructure.
- Capacity point: Peak flow rate achieved before system overload begins.
- Congested regime: High density and declining speed, signaling traffic breakdown.
Effective optimization involves maintaining operations near the capacity point to maximize throughput without triggering instability.
- Monitor flow changes using real-time sensors.
- Adjust traffic signal cycles to avoid reaching oversaturation.
- Implement dynamic speed limits to prevent flow collapse.
Density (veh/km) | Speed (km/h) | Flow Rate (veh/h) |
---|---|---|
20 | 100 | 2000 |
40 | 70 | 2800 |
80 | 30 | 2400 |
Analyzing Lane-Changing Behavior in Multi-Lane Traffic Systems
Lane-changing behavior in multi-lane traffic systems plays a crucial role in understanding how vehicles interact and flow within complex road networks. This behavior can significantly affect traffic conditions, influencing congestion, travel time, and safety. By studying how and why drivers change lanes, researchers can develop models to predict traffic patterns and optimize road designs. These models help identify the key factors that influence lane-changing decisions, such as vehicle speed, traffic density, and road conditions.
One important aspect of lane-changing behavior is the decision-making process, which is influenced by various internal and external factors. Drivers must assess the available gaps in adjacent lanes, the speed of other vehicles, and the potential risks of making a move. Understanding these decisions can provide insights into how to improve traffic flow and reduce bottlenecks on multi-lane highways.
Key Factors Influencing Lane-Changing
- Vehicle Speed: Drivers are more likely to change lanes when they perceive an opportunity to travel faster than the current lane's speed.
- Traffic Density: High traffic density can reduce the likelihood of successful lane changes due to limited gaps between vehicles.
- Driver Intentions: Aggressive or cautious driving styles impact the frequency and safety of lane changes.
- Road Geometry: Curves, intersections, and other road features can influence a driver's ability and desire to change lanes.
Lane-Changing Decision Model
- Identify gaps in adjacent lanes based on vehicle speed and density.
- Evaluate the risk of a collision or disruption in traffic flow from changing lanes.
- Make a decision based on a trade-off between speed gains and potential risks.
- Execute the lane change if the perceived benefit outweighs the risks.
Understanding the dynamics of lane-changing decisions is key to improving traffic management and road safety. Research into this behavior provides a foundation for developing intelligent transportation systems.
Traffic Flow and Lane-Changing Impact
Factor | Effect on Traffic Flow |
---|---|
Frequent Lane Changes | Increases traffic variability and may lead to congestion. |
Smooth Lane Changes | Enhances overall flow and reduces travel time. |
Blocking of Lane Changes | Can cause bottlenecks and increase delays. |
Integrating Traffic Flow Principles into Intelligent Transportation Systems
Understanding traffic flow is a key element in designing and optimizing Intelligent Transportation Systems (ITS). By utilizing traffic flow models, ITS can dynamically manage vehicle movement, improving traffic efficiency and reducing congestion. These systems incorporate real-time data to adapt to changing traffic conditions, thereby facilitating smoother transportation and enhancing road safety.
The integration of traffic flow theories into ITS involves several key principles, such as capacity management, signal optimization, and adaptive routing. These principles enable a system to predict, control, and influence traffic behavior, ensuring that the infrastructure can meet demand without causing delays or accidents.
Core Components of Traffic Flow Integration
- Data Collection: Real-time monitoring of traffic volumes and speeds using sensors, cameras, and GPS data.
- Traffic Signal Control: Adjusting light timings based on traffic density to minimize wait times.
- Dynamic Routing: Providing alternative routes to drivers to alleviate congestion on main roads.
- Incident Management: Detecting and responding to accidents or breakdowns to prevent further disruption.
Key Benefits:
By integrating traffic flow principles, ITS can reduce travel time, enhance fuel efficiency, and improve safety on the roads.
Challenges in Integration
- Data accuracy and reliability of traffic sensors can affect system performance.
- Managing high traffic volumes in urban areas requires advanced algorithms and infrastructure.
- Ensuring compatibility between different traffic management technologies and devices is crucial for smooth operation.
Impact on Future Mobility:
Factor | Effect on Traffic Flow |
---|---|
Real-time Traffic Updates | Improves route planning and reduces delays. |
Adaptive Signal Systems | Optimizes traffic light patterns based on real-time demand. |
Vehicle-to-Infrastructure Communication | Enhances coordination between vehicles and traffic systems for smoother flow. |