Collaborative Artificial Intelligence
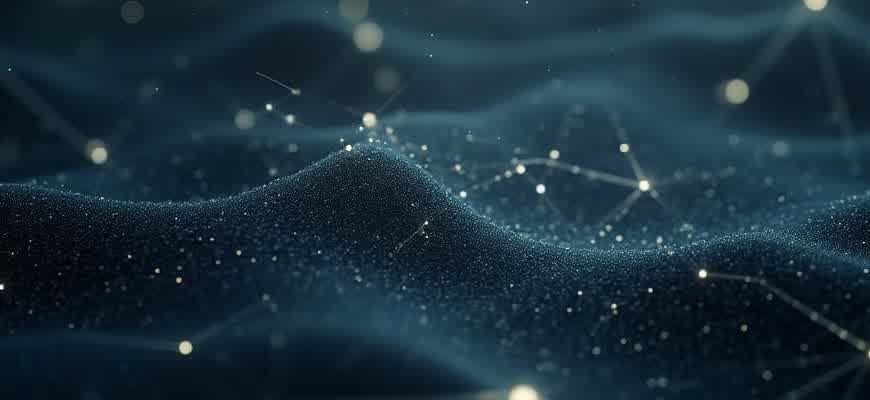
Collaborative artificial intelligence refers to the integration of multiple AI systems working together to solve complex problems that single systems might struggle with. This cooperation enables enhanced decision-making, problem-solving, and innovation by combining the strengths of various AI technologies, such as machine learning, natural language processing, and computer vision.
The concept of collaboration in AI is often structured into several components:
- Data Sharing: AI systems share datasets for improved analysis and learning.
- Task Delegation: Each AI specializes in specific tasks, optimizing performance across a system.
- Mutual Learning: AI agents learn from each other's experiences to continually improve outcomes.
"The power of collaborative AI lies in its ability to harness diverse perspectives and capabilities, enabling more efficient and accurate solutions."
Several practical applications demonstrate the effectiveness of this approach:
Application | AI Roles | Benefits |
---|---|---|
Healthcare Diagnostics | Image analysis, data prediction, pattern recognition | Improved accuracy in diagnoses, faster processing times |
Autonomous Vehicles | Navigation, decision-making, hazard detection | Increased safety, better traffic flow management |
How Collaborative AI Improves Group Decision-Making
Collaborative AI tools have significantly transformed how teams approach decision-making by integrating advanced algorithms into group discussions. These systems provide data-driven insights, allowing teams to make more informed, unbiased decisions. AI's ability to process large volumes of information quickly can enhance team dynamics by presenting relevant patterns and trends that may not be immediately obvious to human members. As a result, decision-makers can leverage AI's analytical capabilities to refine their judgment and reduce cognitive biases.
In team-based environments, the collaborative nature of AI fosters an environment where members can share, discuss, and evaluate ideas more effectively. By incorporating machine learning, natural language processing, and predictive analytics, AI helps streamline communication and improves consensus-building. Collaborative AI does not replace human decision-makers but acts as a support system that augments their capabilities, enabling teams to make decisions faster and with greater accuracy.
Benefits of Collaborative AI in Team Decisions
- Enhanced Data Processing: AI tools can analyze vast datasets in real time, providing teams with up-to-date insights that inform decision-making.
- Bias Reduction: AI helps to identify and mitigate human biases by offering objective recommendations based on facts and data.
- Increased Efficiency: Automated tasks and recommendations free up team members' time, allowing them to focus on higher-level decision-making.
How Collaborative AI Supports Decision-Making
- Data Collection: AI systems gather and aggregate relevant data from various sources, helping teams to make decisions based on comprehensive information.
- Analysis and Prediction: AI algorithms predict potential outcomes based on historical data and trends, offering actionable insights to guide team choices.
- Scenario Simulation: AI can simulate various scenarios to show the impact of different decisions, allowing teams to evaluate possible outcomes before acting.
"Collaborative AI provides a unique opportunity for teams to leverage technology in ways that complement human intuition, enhancing decision quality and overall team performance."
Examples of Collaborative AI Tools
AI Tool | Functionality |
---|---|
IBM Watson | Helps teams analyze large datasets and generate insights for more informed decision-making. |
Google AI | Facilitates real-time collaboration by offering predictive analytics and automation for task management. |
Microsoft Azure AI | Offers machine learning models to assist in decision-making and provides a platform for building custom AI applications. |
Enhancing Global Teamwork through AI-Powered Real-Time Collaboration
Artificial intelligence is transforming the way global teams collaborate in real time. By integrating AI tools into communication and project management systems, companies are enabling smoother, more efficient interactions across time zones and geographical locations. AI-powered solutions can bridge language barriers, automate repetitive tasks, and offer real-time data analysis, thus enhancing productivity and decision-making in remote team settings.
One of the major advantages of AI in global collaboration is its ability to provide instant feedback and recommendations. Whether through smart assistants or collaborative platforms, AI can assist team members in tracking progress, resolving issues, and suggesting optimizations in workflows. This capability ensures that teams stay aligned and informed, even when working remotely or in different time zones.
AI Capabilities for Global Collaboration
- Real-Time Language Translation: AI tools like real-time translators allow team members from different linguistic backgrounds to communicate seamlessly without delays.
- Automated Task Management: AI can assign tasks, monitor progress, and send reminders to ensure that no deadline is missed.
- Data-Driven Insights: AI algorithms analyze performance metrics, providing actionable insights to optimize team productivity and collaboration.
For effective collaboration, it is essential to integrate these AI tools within a unified system that all team members can access. The integration allows teams to operate more efficiently without switching between different platforms. Here’s an example of how different AI-powered solutions can be structured for optimal collaboration:
AI Tool | Functionality | Benefit |
---|---|---|
Real-Time Translator | Translates messages instantly | Enables smooth communication across languages |
Task Automation | Assigns, tracks, and prioritizes tasks | Reduces manual effort and prevents task overload |
Data Analytics | Monitors progress and generates reports | Improves decision-making with data-driven insights |
"AI not only accelerates decision-making but also facilitates a more inclusive, collaborative work environment that bridges gaps between cultures and time zones."
Integrating Collaborative AI into Existing Business Workflows
Incorporating collaborative artificial intelligence into established business processes can significantly enhance productivity and decision-making. By utilizing AI tools designed for teamwork, businesses can streamline communication, improve data processing, and automate routine tasks, enabling human employees to focus on more strategic functions. However, the integration process requires careful planning to ensure that AI systems align with the existing structure and goals of the organization.
The successful adoption of collaborative AI involves more than just deploying new technology. It requires an understanding of the current workflows, employee roles, and the overall business strategy. AI tools must be customized to fit into the existing ecosystem and should enhance the team's capabilities rather than replace human interaction entirely. Below are key steps and considerations for integrating collaborative AI into current business operations:
Steps for Integration
- Assess Existing Workflows: Begin by identifying areas where AI can complement or automate tasks that currently demand significant human effort.
- Choose the Right Tools: Select AI systems that offer features specifically designed to enhance collaboration, such as communication platforms, data analysis tools, or project management assistants.
- Training and Adaptation: Ensure employees are trained on how to effectively work alongside AI systems to maximize productivity.
- Continuous Monitoring and Feedback: Regularly evaluate the performance of AI tools and gather feedback from employees to optimize their effectiveness.
Challenges and Solutions
While AI presents numerous opportunities, integrating it into a business environment can also introduce challenges. These can include resistance from employees, data privacy concerns, and the need for technical expertise to maintain AI systems.
Challenge | Solution |
---|---|
Employee Resistance | Offer comprehensive training and emphasize AI as a tool for collaboration, not replacement. |
Data Privacy | Implement robust security measures and ensure transparency in how data is handled. |
Technical Expertise | Partner with AI vendors for technical support and ensure ongoing staff training. |
Key Takeaway: Successful integration of collaborative AI requires a tailored approach that focuses on complementing human capabilities rather than replacing them. With the right planning and support, AI can significantly enhance business workflows.
How Collaborative AI Enhances Knowledge Sharing and Problem Solving
Collaborative Artificial Intelligence (AI) has significantly transformed the way knowledge is shared and challenges are addressed across various industries. By integrating human expertise with AI capabilities, the collaborative model allows for seamless communication and joint problem-solving, which amplifies the strengths of both parties. This fusion of human and machine intelligence enables more efficient decision-making and accelerates innovation. Furthermore, it opens new possibilities for individuals and organizations to solve complex problems together in ways that were previously unattainable.
Through collaborative AI, data and insights can be exchanged effortlessly between different systems, experts, and teams. The AI acts as a facilitator, organizing and synthesizing information in a way that makes it easier to share knowledge. This dynamic partnership also encourages diverse perspectives, as the AI model can analyze vast amounts of data from various sources, making it possible to uncover hidden patterns and solutions that might otherwise be missed.
Key Advantages of Collaborative AI in Knowledge Sharing
- Real-time collaboration: Collaborative AI enables users to share data and insights instantly, eliminating delays and increasing productivity.
- Information synthesis: AI systems can process and consolidate large amounts of information, ensuring that team members have access to relevant and accurate knowledge.
- Enhanced decision-making: By analyzing vast datasets, AI helps identify patterns that human experts might overlook, contributing to better-informed decisions.
How Collaborative AI Solves Complex Problems
- Data-driven problem identification: AI models can quickly identify issues by analyzing large datasets, providing teams with a clear understanding of the problem.
- Collaborative brainstorming: With AI suggesting potential solutions based on historical data and trends, human experts can collaborate to refine and implement the best approach.
- Continuous learning: As AI systems gather more data and feedback, they continuously improve, adapting to new problems and offering innovative solutions over time.
"Collaborative AI fosters a synergistic relationship where both human and machine capabilities are optimized for greater efficiency and problem-solving potential."
Collaborative AI's Role in Cross-Disciplinary Problem Solving
Discipline | AI's Contribution |
---|---|
Healthcare | AI assists in diagnosing diseases by analyzing medical data, helping doctors make faster, more accurate decisions. |
Finance | AI models predict market trends and identify investment opportunities by processing vast amounts of financial data. |
Engineering | AI enhances design processes by running simulations and offering optimization strategies based on historical data and current variables. |
Enhancing Customer Support with Collaborative AI Solutions
Customer service has evolved significantly with the integration of AI technologies, and the next step in this evolution is collaborative AI. By combining the strengths of human expertise and AI's ability to analyze vast amounts of data, businesses can create more personalized, efficient, and responsive customer service experiences.
Collaborative AI solutions empower agents by automating routine tasks, offering insights, and assisting in complex problem-solving. This synergy results in higher productivity, reduced resolution times, and improved customer satisfaction.
Key Advantages of Collaborative AI in Customer Service
- Faster Response Times: AI chatbots can handle common inquiries, allowing human agents to focus on more complex issues.
- 24/7 Availability: AI-powered systems can provide round-the-clock support without human intervention.
- Personalized Interactions: AI can analyze customer data and recommend tailored solutions, enhancing the overall experience.
- Seamless Handoff: When AI encounters a complex problem, it can quickly transfer the interaction to a human agent with full context.
Collaborative AI Process in Action
- Customer Inquiry: The customer reaches out via chatbot or voice assistant.
- AI Assistance: The AI provides instant responses to common issues, pulling from knowledge bases or FAQs.
- Agent Handoff: If the inquiry is too complex, the AI transfers the case to a human agent, along with relevant data for quick resolution.
- Post-Interaction Analysis: AI reviews the interaction for patterns and suggests improvements for future cases.
Challenges to Consider
Challenge | Potential Solution |
---|---|
Data Privacy | Ensure AI solutions comply with regulations such as GDPR and implement strong data protection measures. |
Customer Trust | Provide transparency on how AI systems work and the role of human agents in the process. |
Integration with Existing Systems | Implement AI solutions gradually and ensure compatibility with current customer service platforms. |
"Collaborative AI bridges the gap between human expertise and machine efficiency, creating a seamless customer service experience."
Data Privacy Considerations When Implementing Collaborative AI
As artificial intelligence becomes more collaborative, concerns regarding the privacy of sensitive data are growing. The integration of machine learning models across various parties, such as different organizations or users, often requires the sharing of large datasets. However, this data can include personally identifiable information (PII), confidential business details, or other private content, posing potential risks if not properly handled. Ensuring that data privacy is maintained while enabling collaboration is a critical challenge in the development and deployment of collaborative AI systems.
When multiple entities share data, managing who can access it and how it is used becomes paramount. With advanced AI models relying on vast amounts of data to function effectively, it is crucial to strike a balance between utilizing this data for training and safeguarding the privacy of individuals and organizations. The following points outline key considerations to address privacy concerns in collaborative AI settings:
- Data Anonymization: Data should be anonymized or pseudonymized before sharing to remove personally identifiable elements.
- Access Control: Strict protocols for controlling who can access the data and the AI model’s outputs must be implemented.
- Transparency: Users should be informed about how their data is being used and have the option to opt out of data sharing when necessary.
Another critical aspect involves compliance with global data protection regulations, such as the General Data Protection Regulation (GDPR) in Europe or the California Consumer Privacy Act (CCPA). Organizations working with collaborative AI must ensure they adhere to these legal frameworks to avoid potential penalties or loss of trust. The following table highlights some of the key privacy requirements from these regulations:
Regulation | Key Privacy Requirements |
---|---|
GDPR | Data minimization, right to access, right to be forgotten, data breach notifications |
CCPA | Right to know, right to delete, right to opt out of data sale |
Important: Collaborative AI systems should incorporate robust encryption methods, both for data in transit and at rest, to ensure that unauthorized parties cannot access or tamper with sensitive information.
Assessing the Value of Collaborative AI Tools in Organizational Settings
When implementing collaborative artificial intelligence tools within a company, it is essential to measure their return on investment (ROI). The effectiveness of these systems can significantly impact productivity, decision-making, and employee satisfaction. Evaluating ROI goes beyond just the financials; it involves understanding how AI tools enhance collaboration, streamline workflows, and reduce operational inefficiencies. With proper measurement, organizations can justify their AI investments and make data-driven decisions regarding tool adoption and scaling.
Measuring the impact of AI-driven collaboration tools requires a comprehensive approach. Several metrics and methods should be considered to gauge both the tangible and intangible benefits. These include time savings, improved team performance, and innovation acceleration. Below, we outline key factors that contribute to evaluating ROI and the tools that can be used for assessment.
Key Metrics for Evaluating Collaborative AI ROI
- Efficiency Gains: Time saved in performing routine tasks and process automation.
- Employee Engagement: The level of user adoption and interaction with the AI tools.
- Cost Reduction: The reduction in overhead and operational costs as a result of AI integration.
- Innovation Impact: The speed at which new ideas and solutions are generated within teams.
Example Metrics for ROI Measurement:
Metric | Before AI | After AI | Difference |
---|---|---|---|
Time to Complete Task | 10 hours | 6 hours | 40% reduction |
Employee Collaboration Score | 70% | 85% | +15% |
Operational Costs | $50,000/month | $40,000/month | $10,000 saved |
"The true measure of ROI lies not only in cost reduction but also in how AI tools facilitate innovation and empower teams to collaborate more effectively."
Strategies for Improving ROI
- Regular Training: Ensure employees are trained to use the AI tools effectively to maximize adoption.
- Customization: Tailor the AI tools to meet specific organizational needs and workflows.
- Continuous Feedback: Collect feedback regularly from users to identify areas of improvement.
- Integration: Seamlessly integrate AI tools with existing systems and workflows to reduce friction.
Training and Onboarding Teams to Use Collaborative AI Tools
Introducing artificial intelligence tools into a collaborative environment requires a comprehensive training strategy to ensure effective usage. Successful onboarding involves familiarizing team members with AI capabilities, guiding them through system features, and fostering an understanding of how these tools can improve workflows. Training sessions should focus on real-world applications and offer hands-on experience to create confidence in using AI-driven solutions. Additionally, providing access to detailed resources will enable teams to continue learning as they integrate these tools into their routine operations.
To facilitate this process, it's important to establish clear learning objectives and incorporate continuous feedback. This approach helps to identify gaps in understanding and adjust training content accordingly. A successful strategy not only involves technical training but also encourages a collaborative mindset, highlighting how AI can enhance team cooperation and decision-making.
Effective Onboarding Steps
- Understand the Tool’s Capabilities: Ensure that team members are familiar with the AI tool’s basic functions and features.
- Practice with Scenarios: Use industry-specific examples to help users understand the tool's application in everyday tasks.
- Provide Access to Support: Set up a support system for troubleshooting and answering questions post-training.
- Ongoing Learning: Encourage continuous improvement by offering follow-up workshops and updated materials.
Key Information to Include in Training Materials
Providing a structured training manual with visual aids, step-by-step guides, and FAQs will enhance the learning experience. Users should also be encouraged to experiment with the tools and share insights with their colleagues.
Training Element | Description |
---|---|
Tool Introduction | Overview of AI features and how they integrate into the team’s workflow. |
Practical Application | Simulations or case studies to apply AI in specific team projects. |
Feedback & Evaluation | Gathering feedback to understand areas for improvement and addressing challenges. |