Z-learning Zeta
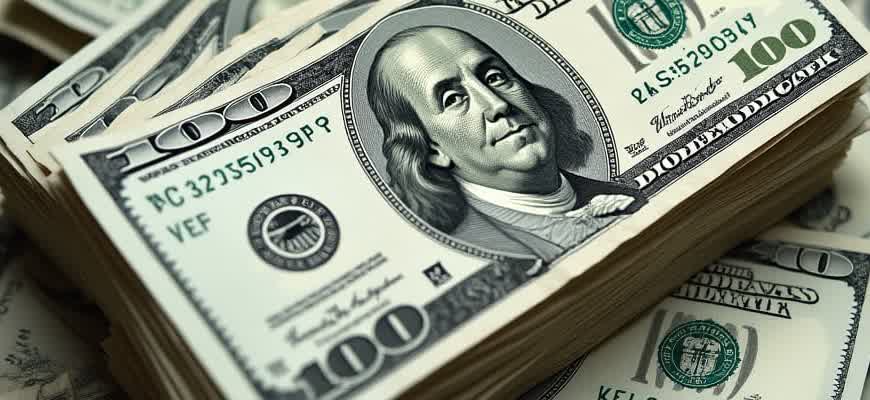
Z-learning Zeta is an advanced methodology within the realm of machine learning that focuses on improving the learning process through dynamic adaptation and efficient decision-making models. This approach leverages both traditional reinforcement learning techniques and modern neural networks to enhance training efficiency and performance outcomes. By fine-tuning specific parameters and utilizing real-time data feedback, Z-learning Zeta seeks to achieve faster convergence while minimizing computational overhead.
The primary objective of this technique is to maximize decision accuracy while minimizing the complexity of the model. Z-learning Zeta introduces several key features that distinguish it from other learning paradigms:
- Adaptive learning rate adjustments based on real-time error analysis
- Optimized use of reinforcement learning combined with neural network architectures
- Reduction of overfitting through integrated regularization strategies
Key Benefits:
Benefit | Description |
---|---|
Efficiency | Significant reduction in training time due to faster convergence rates. |
Scalability | Capability to handle large datasets without sacrificing model performance. |
Flexibility | Can be applied across various domains, including autonomous systems, finance, and healthcare. |
"Z-learning Zeta is a breakthrough in how we approach machine learning tasks, offering both adaptability and precision in complex environments."