Collaborative A.i. Responsibility Lab
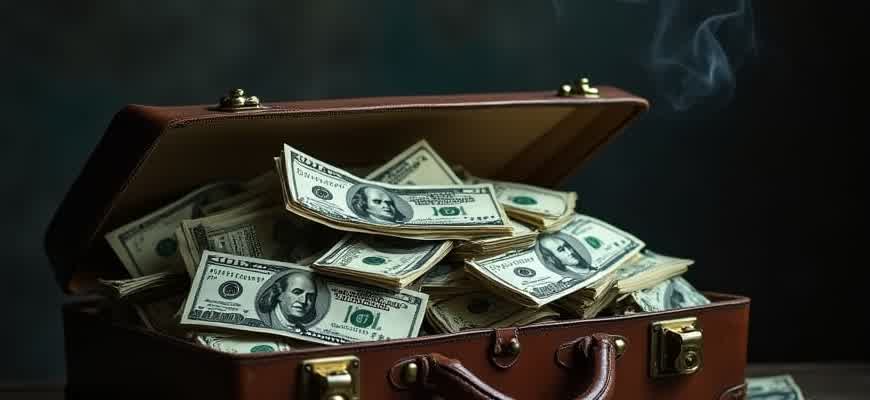
Artificial intelligence (AI) systems are becoming increasingly integrated into decision-making processes across various sectors. As their influence expands, it is crucial to ensure these systems are developed and used responsibly. A key concept emerging in AI development is the idea of a shared responsibility in the creation and deployment of intelligent systems, often referred to as the Collaborative AI Responsibility Framework.
This framework encourages cooperation between developers, organizations, and regulatory bodies to ensure AI operates ethically, safely, and in alignment with societal values. A few critical points in this framework include:
- Ensuring transparency in AI decision-making processes
- Maintaining accountability for AI-generated outcomes
- Promoting fairness in AI applications to avoid biases
The framework also emphasizes the importance of continuous collaboration between stakeholders through structured approaches. These approaches can be summarized as:
- Policy Integration: Harmonizing AI regulations with current laws to ensure legal and ethical consistency.
- Continuous Monitoring: Ongoing oversight of AI systems to detect and mitigate unintended consequences.
- Public Engagement: Involving communities in discussions about the risks and benefits of AI technology.
"Collaboration between diverse stakeholders is essential to establish an ecosystem of trust and accountability in AI."
By establishing these collective guidelines, the goal is to foster AI systems that prioritize human well-being while minimizing harmful effects and ensuring equitable outcomes.
Driving Innovation and Accountability through Collaborative AI Responsibility Labs
The concept of a "Collaborative AI Responsibility Lab" is an emerging framework that aims to bridge the gap between technological innovation and societal responsibility. As AI systems become more integrated into daily life, the need for structured environments to address ethical concerns and ensure accountability grows. These labs provide a space where researchers, developers, and policymakers work together to design AI solutions that are not only cutting-edge but also ethically sound and transparent.
By fostering a culture of collaboration, these labs help ensure that the rapid advancements in artificial intelligence are balanced with thoughtful governance and accountability. In this environment, diverse stakeholders can contribute their expertise, creating solutions that are not just effective but also socially responsible, thus preventing unintended negative consequences on society.
Key Elements of Collaborative AI Responsibility Labs
- Cross-Disciplinary Collaboration: Involving AI developers, ethicists, legal experts, and community stakeholders to address a wide array of challenges in AI deployment.
- Transparency in Decision-Making: Ensuring that the processes used in AI design and deployment are open and understandable to all involved parties.
- Accountability Mechanisms: Establishing clear protocols for AI governance that ensure actions can be traced back to responsible entities.
Methods for Achieving Responsible AI Innovation
- Ethical Guidelines Development: Establishing a shared understanding of the ethical standards that should guide AI development.
- Inclusive Design Thinking: Incorporating diverse perspectives to prevent bias and ensure fairness in AI systems.
- Continuous Monitoring: Regularly evaluating the impact of AI systems on society and making necessary adjustments based on feedback.
Collaborative Frameworks for Accountability
Stakeholder | Role in the Lab |
---|---|
AI Developers | Creating algorithms and systems based on ethical guidelines. |
Ethicists | Advising on the ethical implications of AI systems. |
Policy Makers | Providing regulatory oversight and ensuring compliance with laws. |
Community Representatives | Voicing concerns and ensuring the inclusivity of AI systems. |
"A responsible approach to AI development is not just about creating smarter systems; it's about building trust and ensuring the technology serves everyone equitably."
Optimizing Data Governance for A.I. Systems in Collaborative Environments
In collaborative environments where multiple stakeholders contribute to A.I. systems, optimizing data governance is crucial for ensuring that the data is used responsibly, ethically, and transparently. Proper data management practices are key to safeguarding the integrity and quality of data, which directly impacts the performance and fairness of A.I. models. As these systems evolve through collective inputs, it becomes essential to have clear frameworks in place that can handle complex data-sharing protocols and privacy concerns across diverse entities. This involves setting up structured policies to address data access, ownership, and accountability, all while maintaining compliance with legal standards.
Effective governance for A.I. systems also requires continuous monitoring of data flow, usage patterns, and outcomes in a collaborative setting. A robust governance model should be flexible enough to adapt to new challenges and incorporate feedback from all parties involved. Furthermore, ensuring that the data remains consistent, reliable, and free from bias is a key responsibility for all contributors. To achieve this, a well-organized governance framework must address both technical and ethical considerations, providing a balance between innovation and responsibility.
Key Aspects of Data Governance in Collaborative A.I. Systems
- Data Ownership and Access: Establishing clear ownership rights and access levels for all parties involved.
- Privacy and Security: Ensuring compliance with privacy laws, such as GDPR, and securing sensitive information through encryption and access controls.
- Accountability: Defining who is responsible for data management, quality, and accuracy in the system.
- Bias Mitigation: Implementing strategies to identify and mitigate bias in both the data and the A.I. models.
Steps for Optimizing Governance
- Establish Data Policies: Develop clear, concise policies that define how data will be handled, shared, and governed across all stakeholders.
- Implement Data Quality Standards: Set benchmarks for data accuracy, consistency, and completeness, ensuring that all contributors adhere to these standards.
- Monitor Data Usage: Continuously track and audit data usage to ensure compliance with governance policies and identify any irregularities.
- Foster Transparent Communication: Create communication channels for stakeholders to discuss issues, share feedback, and update data governance protocols as needed.
Collaborative Data Governance Model
Aspect | Collaborative Approach |
---|---|
Data Sharing | Defined roles and permissions for all contributors to access and share data. |
Transparency | Clear, open communication regarding how data is used, processed, and protected. |
Ethical Oversight | Independent review bodies to ensure compliance with ethical standards and bias reduction. |
"The success of collaborative A.I. systems depends not only on technological advances but also on how well the data governance models are structured and implemented."
Establishing Clear Metrics for AI Impact and Responsibility
In order to ensure the ethical development and use of AI technologies, it is crucial to define measurable criteria that accurately assess their impact on society. These metrics must not only evaluate the performance of the AI systems but also consider broader social, environmental, and economic consequences. Establishing clear standards helps in determining whether AI applications align with human values and legal frameworks, providing transparency and accountability in AI's deployment across industries.
Transparent metrics allow stakeholders–including developers, policymakers, and the public–to track the effects of AI over time. By creating these measurement systems, the tech community can ensure that AI serves the common good while minimizing harm. Below are key elements that should be part of any AI impact and responsibility assessment.
Key Metrics for AI Accountability
- Fairness and Bias: Tracking AI's ability to avoid biased decision-making processes and ensuring equitable outcomes for all groups.
- Environmental Impact: Measuring the energy consumption, resource usage, and carbon footprint of AI models, especially large-scale deep learning systems.
- Data Privacy: Evaluating how well AI systems handle personal data, ensuring compliance with privacy regulations and protecting individuals' rights.
- Transparency: Assessing the clarity of AI decision-making processes and ensuring that stakeholders can understand how and why decisions are made.
- Accountability: Identifying clear responsibilities for developers and organizations when AI systems cause harm or malfunction.
Tools for Measuring AI Accountability
- Impact Assessment Frameworks: These frameworks help measure the societal and ethical implications of AI systems before deployment, allowing for the identification of potential risks.
- Real-time Monitoring Systems: Tools that continuously track AI behavior in the real world to ensure compliance with ethical standards and detect anomalies or harmful outcomes.
- Independent Audits: Regular audits conducted by third-party organizations to evaluate AI performance against established ethical standards.
Example of AI Impact Metrics in Practice
Metric | Measurement Method | Purpose |
---|---|---|
Bias Detection | Regular testing with diverse data sets | Ensure fairness across demographic groups |
Energy Consumption | Tracking power usage and carbon footprint | Minimize environmental impact |
Data Privacy Compliance | Audit of data protection practices | Protect individual privacy rights |
“The success of AI accountability depends not only on creating effective metrics but also on fostering a culture of transparency and shared responsibility within the development community.”
Designing AI Models with Bias Mitigation at the Core
Creating artificial intelligence systems that are both effective and fair requires deliberate integration of bias mitigation strategies into the design process. The risks associated with AI bias are not only ethical but can lead to harmful social and economic consequences. Developers must consider both technical and social factors when addressing biases, ensuring that AI systems work fairly across diverse demographic groups and avoid amplifying existing disparities.
To achieve this, the development process should center on three critical stages: identification of potential biases, incorporation of mitigation techniques, and continuous evaluation. Each stage requires a unique set of tools and methodologies, including the use of diverse training data, algorithmic fairness metrics, and ongoing auditing of system outputs.
Key Strategies for Bias Mitigation
- Data Diversification: Ensuring that the dataset is representative of all relevant populations to prevent skewed training data.
- Bias Audits: Regularly auditing both input data and model outputs to detect and correct biases in real-time.
- Algorithmic Transparency: Designing algorithms in a way that allows for easier identification and correction of biased patterns.
- Stakeholder Involvement: Including input from diverse groups during the design and testing phases to ensure broad perspectives are considered.
Important Considerations
Bias mitigation is not a one-time fix but an ongoing process. Developers must prioritize continuous monitoring and updates to AI systems to ensure they evolve in line with changing social contexts and fairness standards.
Comparison of Bias Mitigation Techniques
Technique | Impact | Challenges |
---|---|---|
Preprocessing Data | Reduces bias in the training dataset | Requires significant data modification |
In-Processing Modifications | Adjusts model behavior during training | Complex to implement without sacrificing model performance |
Post-Processing Adjustments | Corrects output decisions to ensure fairness | Can be less effective if biases are deeply embedded |
Establishing Ethical Guidelines for A.I. Integration in Cross-Industry Projects
As artificial intelligence becomes more integrated into various sectors, it is crucial to develop and implement ethical frameworks that ensure responsible usage. The application of AI in industries such as healthcare, finance, and manufacturing requires careful consideration of both the technological potential and the potential risks. These guidelines should address concerns like fairness, transparency, accountability, and privacy, which are essential for fostering trust among stakeholders and ensuring the long-term success of AI adoption.
Creating a comprehensive ethical framework also involves identifying the specific challenges and requirements of each sector. By doing so, industries can avoid common pitfalls, such as bias in decision-making or data misuse, while promoting innovations that benefit society. Below are several key elements to include in the establishment of ethical standards for AI integration in cross-industry collaborations:
Key Ethical Considerations
- Bias and Fairness: Ensuring that AI systems are trained on diverse, representative data to prevent biased outcomes in decision-making processes.
- Transparency: AI decision-making processes should be understandable and explainable to stakeholders to build trust and prevent misuse.
- Privacy Protection: Maintaining data privacy and safeguarding personal information are essential, especially when AI systems process sensitive or confidential data.
- Accountability: Clear accountability measures must be in place to hold developers and organizations responsible for any harm caused by AI technologies.
AI ethics must consider both the technological advantages and potential societal risks to ensure the responsible development and deployment of AI across industries.
Practical Steps for Ethical AI Deployment
- Establish Ethical Committees: Form cross-industry ethical review boards to evaluate AI integration projects and monitor ongoing compliance with ethical standards.
- Develop Clear Regulations: Draft industry-specific regulations that outline ethical responsibilities and set boundaries for AI usage, ensuring consistent governance across sectors.
- Continuous Monitoring and Auditing: Implement systems for regular audits of AI systems to assess their performance and compliance with ethical standards, adjusting as necessary.
- Stakeholder Involvement: Engage diverse stakeholders, including regulatory bodies, advocacy groups, and the public, in discussions on AI development and deployment to ensure broad consensus on ethical concerns.
Examples of Industry Guidelines
Industry | Key Ethical Concern | Guideline |
---|---|---|
Healthcare | Patient Privacy | Implement strict data anonymization protocols and ensure compliance with health data regulations (e.g., HIPAA). |
Finance | Fair Lending Practices | Ensure AI models do not discriminate based on race, gender, or socioeconomic status in credit decision-making. |
Manufacturing | Workplace Safety | AI systems must be rigorously tested to prevent accidents or malfunctions that could endanger human workers. |
Developing Real-Time Monitoring Systems to Track A.I. Decisions in Collaborative Environments
In the development of artificial intelligence systems for collaborative environments, it becomes crucial to ensure transparency in AI decision-making processes. Real-time monitoring systems offer a solution to track the actions of AI models, making it easier to assess their behavior and outcomes. These systems help in understanding how AI interacts with human partners and influences joint decisions, allowing for quick identification of potential issues that may arise during collaboration.
To implement such systems effectively, one must focus on integrating monitoring tools that capture data continuously, providing insights into the reasoning behind AI decisions. A well-structured system should not only collect raw data but also process and present it in a way that is accessible and understandable to human collaborators. This enables teams to maintain control over the process while ensuring accountability.
Key Components of Real-Time Monitoring Systems
- Data Logging – Continuous tracking of AI inputs, outputs, and intermediate states during the collaboration process.
- Transparency Mechanisms – Techniques that allow collaborators to observe and comprehend AI reasoning in real-time.
- Alerting Systems – Automatic notifications triggered by anomalies or deviations from expected behavior.
Challenges in Tracking AI Decisions
- Scalability – As systems grow, real-time monitoring becomes more complex and resource-intensive.
- Data Overload – Managing large volumes of data without overwhelming users can be difficult.
- Interpretability – AI's decision-making logic can often be opaque, making it challenging to trace the reasoning behind its actions.
Approaches to Improve Monitoring
Approach | Benefits | Challenges |
---|---|---|
Model Explainability Techniques | Enhances understanding of AI decisions | Increased computational costs |
Continuous Feedback Loops | Real-time adjustments based on human input | Dependency on human engagement |
Automated Auditing Tools | Efficient tracking and logging of decisions | Risk of missing contextual nuances |
"Real-time tracking systems are essential to ensure that AI collaborators align with human values, preventing unintended consequences during joint decision-making."
Building Trust Between A.I. and Human Stakeholders Through Clear Communication
Effective collaboration between artificial intelligence systems and human stakeholders relies heavily on transparent communication. When the intentions and capabilities of A.I. systems are clearly conveyed to all parties, it fosters an environment of trust and minimizes the potential for misunderstandings. A.I. stakeholders, including developers, users, and regulators, must be kept informed about how the system functions and how decisions are made. This transparency helps prevent errors and ensures that the technology is used responsibly and ethically.
Clear communication serves as the cornerstone for fostering mutual understanding between A.I. systems and human users. By providing accessible explanations of the system's reasoning, capabilities, and limitations, stakeholders can better trust the decisions made by the A.I. and can hold the system accountable when necessary. Such openness encourages continuous feedback, which is essential for improving both the technology and its integration within society.
Key Practices for Transparent Communication
- Clear Documentation: Detailed documentation should be made available to explain how the A.I. system operates, what data it processes, and how it makes decisions.
- Frequent Updates: Stakeholders should receive regular updates on system performance, including any modifications, upgrades, or changes in functionality.
- Accessible Language: Using clear and simple language to explain complex A.I. processes ensures that stakeholders from all backgrounds can understand the technology.
Benefits of Open Communication
- Enhanced Trust: Stakeholders feel more confident in their interactions with A.I. when they understand its actions and decision-making process.
- Reduced Risk of Misuse: Clear communication helps ensure that A.I. systems are used as intended and reduces the potential for harmful unintended outcomes.
- Increased Accountability: When the decision-making process is transparent, it's easier to pinpoint the source of errors or unethical behavior, ensuring accountability.
Communication Strategies for A.I. Developers
Strategy | Impact |
---|---|
Open Source Collaboration | Encourages external review and input, which leads to improved reliability and public confidence. |
Transparent Model Design | Clarifies how algorithms are designed, reducing ambiguity and helping stakeholders trust the system's behavior. |
Real-Time Feedback Loops | Allows continuous improvement of the A.I. system, based on direct user experiences and concerns. |
“Trust is built when both parties understand each other’s roles and intentions. Clear communication bridges the gap between A.I. and human stakeholders, making collaboration smoother and more effective.”
Implementing Risk Management Protocols for AI Failures in Collaborative Systems
When integrating artificial intelligence (AI) into collaborative systems, ensuring the reliability of these systems is essential. In cases of AI malfunction, prompt risk management procedures must be in place to mitigate any potential negative impacts on the system’s users and the broader environment. Failure to do so may lead to data breaches, system failures, or even significant financial losses. It is crucial to establish clear protocols to manage the risks associated with AI failures in collaborative settings, which can involve multiple stakeholders, technologies, and dynamic processes.
To effectively manage these risks, the design and implementation of structured protocols are necessary. These protocols should encompass preventative measures, detection systems, and rapid response mechanisms. This approach minimizes damage and ensures that recovery is swift and efficient, even in the event of system disruption. Below are the key components of such a risk management framework.
Key Elements of an AI Risk Management Protocol
- Risk Identification: Identifying potential failure points within the AI system is critical. These include biases in data, inaccurate algorithms, and system vulnerabilities.
- Impact Assessment: Evaluating the potential consequences of failures, both short and long term, and determining their scope.
- Mitigation Strategies: Developing solutions to reduce the likelihood of failures, including redundancy systems and frequent testing.
- Continuous Monitoring: Establishing real-time monitoring systems that detect and flag potential issues early.
Response and Recovery Procedures
- Automated Fail-safes: Designing the system with fail-safe mechanisms that automatically shut down or correct faulty operations when failures are detected.
- Human Oversight: Ensuring human operators can intervene when automated systems cannot resolve a problem or when critical decisions need to be made.
- Communication Protocols: Establishing clear communication channels for reporting issues and providing real-time updates to stakeholders.
- Post-Incident Analysis: After a failure, conducting thorough investigations to identify root causes and implement improvements to avoid recurrence.
Risk Management Table for AI Collaborative Systems
Risk Type | Impact | Mitigation Strategy |
---|---|---|
Data Bias | Unfair outcomes, loss of trust | Regular auditing, diverse training data |
Algorithmic Errors | Inaccurate decisions, system failure | Frequent testing, transparent algorithms |
Security Vulnerabilities | Data breaches, unauthorized access | Encryption, multi-layer security |
Important: A proactive risk management protocol not only protects the system from failures but also builds trust among users and stakeholders, fostering a more reliable collaborative AI environment.